AI Week In Review 23.09.23
DALL-E 3, Google Bard, Windows CoPilot rolls out, YouTube creation tools, StableAudio and new deep learning architectures challenge transformers
Cover art: Funky AI-generated spiraling medieval village captivates social media.
Top Tools
Google Bard is now an Answer Engine! This week’s latest Google Bard update is interesting and useful enough that we should highlight it as a Top Tool. Here’s why: Google Bard has announced that Bard can connect to extensions, similar to chatGPT plug-ins, to extend including Google Flights, Maps, YouTube, Google Workspace (including drive docs and emails), and more.
I have already found the latest Bard personally useful to me, because it can search my documents and emails out of Google Workspace and act as a smart personal AI summarizer. Bard also accepts voice input, so I can speak and ask for topic-related email summaries, and get quick summaries. Connecting to Google Workspace is available for free to anyone with a Gmail account.
Google Bard also integrates with web search and reference-checking, similar to Microsoft Bing chat. While Bard is still powered by Palm 2, it keeps getting better.
AI Tech and Product Releases
OpenAI announces release of DALL-E 3 to research preview now and availability in October. DALL-E 3 appears to be a huge step up for AI text-to-image generation, able to “generate images from text descriptions with unprecedented fidelity and creativity.”
It is built natively on ChatGPT, so you use ChatGPT as a prompt refiner.
Is shows a big increase in precision in terms of response to specific prompts and image generation resolution over both Dall-E 2 and competitive image generators like Midjourney.
Examples show it can output text in images precisely and correctly.
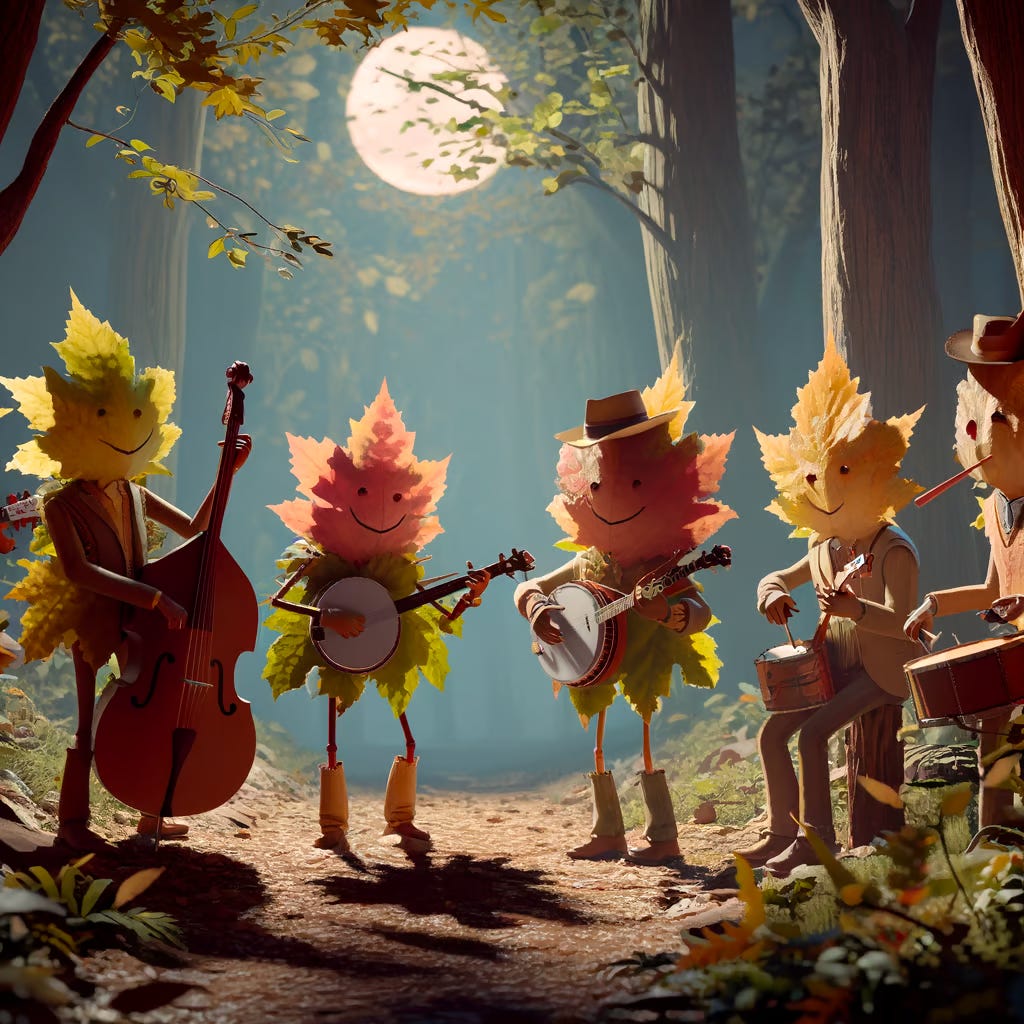
Stability AI has come out with Stable Audio, a music generation product that can produce original AI-generated music in a number of styles. They say “Our mission is to empower creators with tools that aid musical creativity” and mention that users can use outputs as samples in their own music.
Microsoft announced that “Copilot will begin to roll out in its early form as part of our free update to Windows 11, starting Sept. 26 — and across Bing, Edge, and Microsoft 365 Copilot this fall.” This includes”AI-powered” features across the range of tools, and in the OS itself.
YouTube announced a slew of AI-powered tools for creators at its annual Made on YouTube event on Thursday. For example, Dream Screen creates AI-generated videos and photos for the background of YouTube Shorts, and AI dubbing will dub videos into other languages.
AI Research News
Researchers show that “Connecting Large Language Models with Evolutionary Algorithms Yields Powerful Prompt Optimizers” with a framework they call EvoPrompt. This framework leverages LLMs with Evolutionary Algorithms (EAs) to create improved prompts. “EvoPrompt significantly outperforms human-engineered prompts and existing methods for automatic prompt generation by up to 25% and 14% respectively.”
In One Wide Feedforward is All You Need, researchers look at optimizing the transformer architecture by reducing redundancy in the Feed Forward Network (FFN) part of the architecture:
Concretely, we are able to substantially reduce the number of parameters with only a modest drop in accuracy by removing the FFN on the decoder layers and sharing a single FFN across the encoder.
They are able to show substantial gains in both accuracy and latency with their changes compared with original Transformer architecture.
Another improved architecture challenging transformers has been Retentive Network (RetNet). Microsoft and Tsinghua researchers published the paper “Retentive Network: A Successor to Transformer for Large Language Models” on it in July. They introduced “a multi-scale retention mechanism to substitute multi-head attention, which has three computation paradigms, i.e., parallel, recurrent, and chunkwise recurrent representations.”
Now the paper “RMT: Retentive Networks Meet Vision Transformers” - shared on HuggingFace - applies the ResNet idea to vision models, bringing knowledge of distance via an RNN-like decay based on distance to the vision model. The result is “exceptional performance across various computer vision tasks,” where “RMT achieves the highest Top1-acc when models are of similar size and trained with the same strategy.” This can used on vision tasks like object detection and instance and semantic segmentation.
The paper MetaMath: Bootstrap Your Own Mathematical Questions for Large Language Models creates a fine-tuned LLM built for mathematical reasoning:
we start by bootstrapping mathematical questions by rewriting the question from multiple perspectives without extra knowledge, which results in a new dataset called MetaMathQA. Then we fine-tune the LLaMA-2 models on MetaMathQA. Experimental results on two popular benchmarks (GSM8K and MATH) for mathematical reasoning demonstrate that MetaMath outperforms a suite of open-source LLMs by a significant margin. … Particularly, MetaMath-70B achieves an accuracy of 82.3% on GSM8K, slightly better than GPT-3.5-Turbo.
AI Business and Policy
Competition heats up as “OpenAI Hustles to Beat Google to Launch ‘Multimodal’ LLM”.
Is Google looking to drop Broadcom as their supplier of TPU chips? They denied it, but “Broadcom falls on report Google discussed dropping firm as AI chip supplier.”
Meanwhile, Nvidia is on track to become the number one semiconductor company in 2023, with revenues of $53 billion, thanks to the huge demand for their AI chips.
Axios reports the “UN is deadlocked over regulating AI”. My reaction? Good. They mention that “the thorniest question in AI governance is how to involve China” and it would be best to not let drive for global regulation stop progress on AI or lead to giving China leverage on such a regulatory regime.
AI got a lot of mention at UN speeches this month. UK Deputy Prime Minister Dowden said the world must pass ‘AI stress test’: “AI is the biggest transformation the world has known …Our task as governments is to understand it, grasp it, and seek to govern it, and we must do so at great speed.”
Despite the embarrassment of another supermarket whose AI-driven recipe app went awry, a UK supermarket is giving it another go: “Waitrose turns to AI to create recipes for successful food products.”
AI is helping pharmaceutical companies speed up clinical trials: “Major drugmakers are using artificial intelligence to find patients for clinical trials quickly, or to reduce the number of people needed to test medicines, both accelerating drug development and potentially saving millions of dollars.”
AI Opinions and Articles
Alex Kantrowitz asks: “Is Generative AI an Enterprise Thing?” and suggests it is:
About a year into the Generative AI phenomenon, it’s becoming evident that the technology is most useful in the enterprise first, with broader consumer adoption perhaps to follow. … At work, people will have real incentive to learn how to use these products, figure out their prompts, and master their intricacies, especially given that their next promotion, raise, or their job itself might depend on it.
This may be a good thing, as the main utility of generative AI is the automation of a slew of time-consuming yet low-value added ‘white collar’ informational work activities: Writing, analyzing, brainstorming, summarizing, researching, and more. If all AI does is offload the 20% most boring part of our jobs, its a good thing.
A Look Back …
This week, Intel honored Dr. Fei-Fei Li, Professor at Stanford University, for her contributions to Artificial Intelligence. She was also listed as a Top 100 most influential people in AI by TIME magazine.
One of her contributions to AI mentioned in the TIME essay is her work on ImageNet, the database of labelled images used to benchmark image classification ML algorithms:
Born in Chengdu, China, she moved to the U.S. at 15, studied physics and computer science at Princeton, and completed her Ph.D. in electrical engineering at the California Institute of Technology. In 2006, Li began work on ImageNet, a database of images accompanied by text descriptions of their contents. By 2009, Li and her team, with the help of crowd-sourced workers, had labeled 3.2 million images. A year later, they hosted a competition to see who could design an AI system that would most accurately determine the contents of the images. By giving researchers a common benchmark, Li supercharged the development of AI image-recognition systems.