AI Adoption: From Hype to Utility
The AI Adoption Cycle is Evaluate, Pilot, Adopt, Optimize, Standardize. Innovative firms are usefully adopting AI for their benefit, but most businesses are still piloting AI.
Preface
These thoughts on AI adoption began as part of an article on AI for healthcare. However, the AI adoption cycle in healthcare organizations follows similar trajectories to businesses in other industries, so this applies in general business terms. Our final installment of AI for Healthcare is coming soon.
Business Adoption of AI
As we get closer to the 2-year anniversary of the ChatGPT release, the initial AI hype cycle has worn off and use of generative AI across industries is shifting from novelty to real-world use.
Generative AI technology is novel, foundational, and rapidly evolving. Both the technology and how and where to apply it are novel for many organizations and employees. As with any technology, there is an adoption process to go through with AI. Businesses look for returns on any technology adoption or investment, so as we move from AI as novelty to practical use of AI, there is increasing focus on value and achieving ROI.
Suppose you had to answer the question “How can I best use AI to my advantage?” The best advice for either personal or productive business adoption of AI technology would be similar and something like this:
Start by experimenting with AI; try it out to evaluate its capabilities and see what it can do.
Find a ‘low-hanging fruit’ task AI can help with and pilot its use.
As you get more familiar with AI, broaden its application to more valuable use cases.
Adjust your workflows to optimize how you use AI for best productivity.
Finally, standardize on the best practices and uses for applying AI, and automate what you can.
AI technology adoption for most organizations will follow that same pattern, but in a more structured way, going through these five stages of AI technology adoption:
Evaluate: Initial experiment and evaluations of AI to determine what AI can and cannot do; this may be combined with pilot and prototype AI use to try out the technology, experiment, and learn its capabilities. Evaluation and adjustment of use will continue through all stages of adoption.
Pilot: Initial pilot use of AI, trying it out in either a ‘quick win’ simple use case or where it may be most effective.
Adopt: Broaden adoption across high ROI use cases. AI starts to add real value here by displacing more business workflows with a new tool and process.
Optimize: Business processes are adapted and adjusted to make the best use of the new AI technology. Since generative AI is so fundamental to and may be completely overturned and replaced as foundational generative AI becomes inserted into workflows. The optimization may include automation, as whole tasks might be automated with AI by changing the flow to support full automation.
Standardize: Generative AI becomes an invisible part of business processes. Align business processes with AI applications and institutionalize best practices.
The Early Adopters
Where are most businesses today in terms of generative AI adoption? Each business and industry fits on a range in terms of their readiness to adopt AI: The innovators try the latest novel technology on the bleeding edge; early adopters follow close behind; the majority wait to see how others do before diving in. This model of the adoption cycle is shared below.
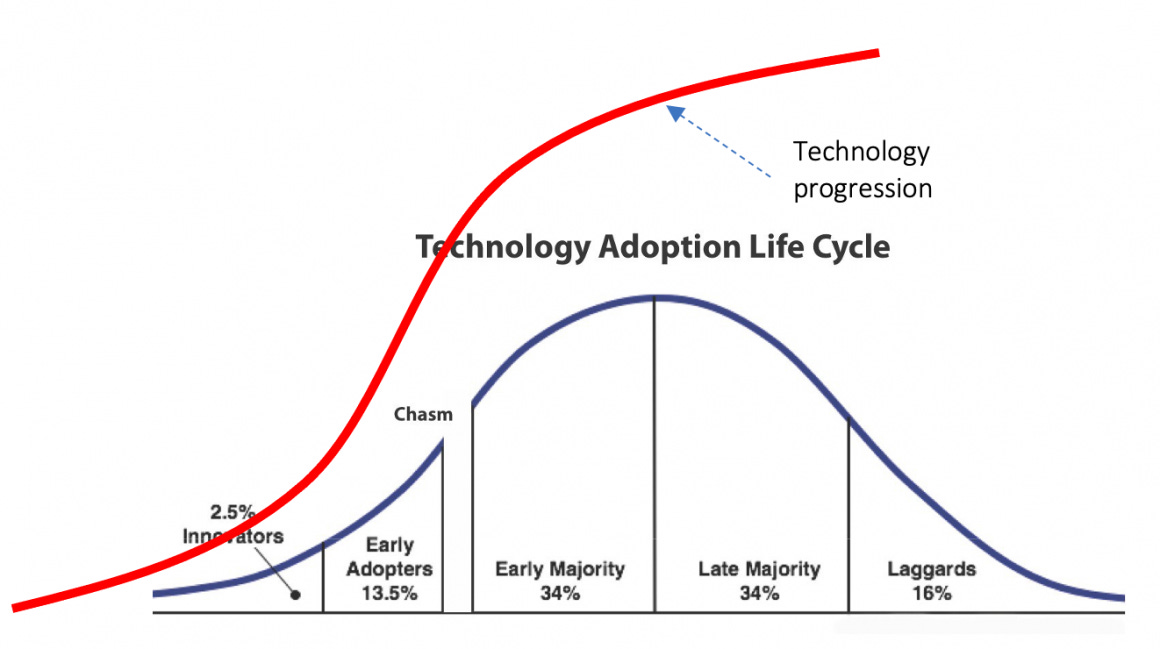
When ChatGPT went viral, it acquired 100 million users in the first few weeks, the steepest adoption ever of a modern technology. One year after ChatGPT hit the market, more than half of companies surveyed by PWC (54%) have implemented GenAI in some areas of their business. However, ChatGPT use is broad but also shallow, used for personal use cases and pilot evaluations, which do not yet impact key business workflows.
Early adoption has been in written communications, customer service and software development, areas where a chatbot or text-based interface and output provide value: Writing assistance for marketing, legal, journalism, and product documentation; coding and software development support; research and brainstorming assistance. In these domains, there have been successful and broad uses by Early Adopters to get AI through Pilot phase and into Adoption phase.
As case in point has been AI coding assistants, the leading-edge application for LLMs since GitHub Copilot. There has been a surge in AI assistants for coding, with dozens of competing AI assistant plug-ins, AI-first editors, and AI software development support application. AI coding tool adoption is widespread by software developers; it was reported in 2023 that 92% of US-based developers were already using AI coding tools. A growing fraction of code is AI-generated.
AI coding assistants have crossed the chasm to mainstream adoption, but they have yet to fully prove themselves. Research from last year found use of AI resulted in “Downward Pressure on Code Quality” and even now there are questions around how much AI actually helps developer productivity. This is getting resolved as AI models and applications improve, and they are improving rapidly.
AI image generation applications are following a similar pattern to language applications based on LLMs. AI image generators were crude toys in 2022, but by 2023 were good enough for use cases such as generating ad copy. Images and designs that required Photoshop before now can be done better with image gen AI integrated into an image editing framework.
Now there are many controls on image output from AI image generation models and ways to control style, branding, resolution, and realism. As the quality of the AI model improves and controls get more precise, the application is migrating from just draft-level use cases to polished, production use cases.
The Value Proposition for AI Adoption
The promise of AI to improve business costs is real and recognized. A McKinsey survey from 2023 found that overall, 42% of respondents said they’d seen reduced costs from use of AI, and 59% claimed increased revenue. (This was reported in Stanford’s 2024 AI index.) AI is most helpful is in service operations (think call centers and communications) and manufacturing, but it holds massive promise across the board.
While there are early adopter functions and innovative organizations, most business organizations and most functions are in the first stages of adoption of generative AI. While ‘innovators’ and ‘early adopters’ are already in the real-use adoption stage for gen AI, the majority (both ‘early majority’ and ‘late majority’) are still in the evaluation and pilot stages. Laggards are not engaged with AI at all yet.
For the majority of businesses and use-cases, more widespread adoption is still to come.
The ‘Sonic Boom’ of AI adoption
One way we know we are currently in the initial stages of generative AI adoption: Most of the AI technology to be adopted hasn’t even been developed yet.
Consider how far we’ve come in AI lately. In the past year, foundation AI models have gone from being text-only LLMs and expanded their modalities to audio and vision, with voice input-output, image, and video understanding. With better reasoning, such as with the o1 model, and function-calling and structured outputs for precision use in AI agentic applications, the generative AI keep expanding.
The next generation of AI models that will arrive in 2025 will be a big step up from what we have now. We have several OOMs (orders of magnitude) of improvement to go in foundation AI models.
At their most recent DevDay, OpenAI mentioned 2 million AI developers that are developing on their AI platform. This extraordinary number of developers are creating many AI applications; most are yet to be released. Tomorrow’s production AI applications will be far more powerful, capable, and varied than what is available now.
The continued scaling of AI makes the technology adoption process an interesting challenge. Organizations that are slow to integrate AI technology may find themselves with obsolete implementations by the time they are done. If they wait for AI to perfect itself, they will be even further behind.
This is the “sonic boom” of AI development: The speed of AI improvement is outpacing the adoption cycle of slower-moving organizations.
The best strategy to counter this is to assume and plan for improvements to AI, by developing flexible AI applications that can be easily or even automatically upgraded as AI improves. Fast movers and adaptive learners will win.
Conclusion
As the generative AI novelty hype dies down, AI capabilities keep marching forward, and both people and organizations look to the best ways of adopting AI to get the most out of it.
Most businesses now recognize the potential benefits of AI, and innovative ‘early adopter’ organizations have incorporated AI already in their flows. Some applications, such as AI coding assistants, have already ‘crossed the chasm’ to mainstream use.
For most businesses in traditional industries, however, typical AI use is still evaluations and pilots. As they plan AI adoption, businesses need to consider specific AI adoption opportunities in terms of risk, complexity, and value. Initial pilots may be the easiest (low complexity) use cases rather than the most potential value, which might require more investment and effort.
One challenge for organizations adopting AI is the problem of building on a moving target as AI improves. Planning for AI advances will be a needed part of AI adoption strategies.
Over the next two to five years, organizations that successfully incorporate AI applications into their workflows will start to reengineer their flow around AI integrations. Generative AI could become invisible to users of their workflows, or alternatively, AI could completely automate the workflow, in effect replacing the workflow.